1-10鍵觸摸等標(biāo)準(zhǔn)品芯片。大量庫(kù)存,現(xiàn)貨供應(yīng)。
服務(wù)/SERVICE11
產(chǎn)品中心/product
觸摸方案/SOLUTIONS
優(yōu)勢(shì)/Advantage
-
獨(dú)立的創(chuàng)意的開(kāi)發(fā)團(tuán)隊(duì)
琪鑫微擁有經(jīng)驗(yàn)豐富的開(kāi)發(fā)團(tuán)隊(duì),專(zhuān)注各類(lèi)產(chǎn)品功能開(kāi)發(fā)定制。
-
完善的項(xiàng)目管控體系
項(xiàng)目進(jìn)程透明化、流程高效化,以規(guī)范化。管控質(zhì)量,縮短開(kāi)發(fā)周期。
-
周到的售后服務(wù)
提供7x12小時(shí)技術(shù)支持、快速解決客戶(hù)難題,解決您的后顧之憂(yōu)。
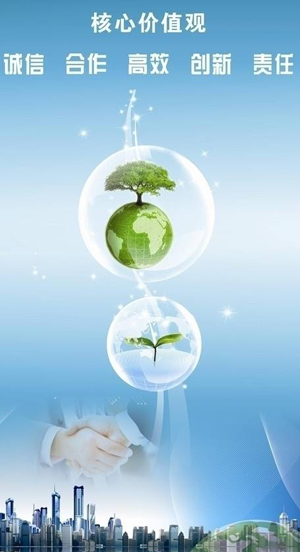
-
強(qiáng)大的供貨能力
我們堅(jiān)持為客戶(hù)提供穩(wěn)定的貨源,備有充足的貨源,足量、及時(shí)供應(yīng)。
-
客戶(hù)技術(shù)資料保密
職業(yè)操守是每個(gè)公司必備的服務(wù)前提,我們公司有著嚴(yán)格的保密制度。
-
不斷提升核心競(jìng)爭(zhēng)力
我們要求每個(gè)員工都要不斷提升自己,保證客戶(hù)與公司始終保持技術(shù)前列。
新聞中心/news

-
20
2022-04觸摸芯片自動(dòng)檢測(cè)環(huán)境功能重要性淺談!
目前,觸摸集成電路,也就是觸摸IC,觸摸芯片或者是觸摸MCU越來(lái)越多地應(yīng)用在電子產(chǎn)品上。觸摸芯片的出現(xiàn)使得電子產(chǎn)品更美觀(guān),更可靠,普遍受到電子產(chǎn)品設(shè)計(jì)者制造商的歡迎?! ≡缙诘挠|摸主要是電阻
-
12
2020-08觸摸臺(tái)燈哪個(gè)值得買(mǎi)?
插電小夜燈觸摸遙控臺(tái)燈 懶人福音設(shè)計(jì),無(wú)限遙控開(kāi)關(guān),親測(cè)3-5米范圍內(nèi)有效,方便實(shí)用,滿(mǎn)足日常所需,5檔亮度可調(diào)。 雷士照明LED護(hù)眼兒童臺(tái)燈觸摸調(diào)光臺(tái)燈 強(qiáng)韌鐵藝主燈體高密度鐵藝燈體,堅(jiān)
-
04
2020-08未來(lái)控制板芯片發(fā)展好的原因!
產(chǎn)品的發(fā)展是隨著市場(chǎng)需求和經(jīng)濟(jì)發(fā)展的不斷推助才能通暢無(wú)阻,當(dāng)然其中其自身的特點(diǎn)也是不容忽視的一個(gè)根本存在??刂瓢逍酒?從問(wèn)世以來(lái)就得到用戶(hù)的不斷追捧,究其原因跟其優(yōu)質(zhì)特性息息相關(guān),不
-
29
2019-10人體觸摸和液體檢測(cè)淺談!
目前各種電子產(chǎn)品的觸摸應(yīng)用,分為觸摸屏和觸摸按鍵兩大類(lèi)。觸摸屏一般應(yīng)用于帶有小規(guī)模顯示屏的智能設(shè)備,觸摸按鍵則應(yīng)用于各種電子設(shè)備或產(chǎn)品上,用來(lái)替代傳統(tǒng)的機(jī)械按鍵。無(wú)論是觸摸屏還是觸摸
-
16
2018-10觸摸芯片高阻輸出和單端模擬電壓輸出對(duì)比
傳統(tǒng)模擬量輸出描述: 一直以來(lái),觸摸芯片在應(yīng)用到有要求模擬量輸出的場(chǎng)合,無(wú)一例外地使用高阻輸出,接分壓電阻的方式來(lái)提供給主控芯片以不同的電壓量。如下圖所示: 這種模擬量輸出的缺點(diǎn),一是
-
16
2018-01觸摸屏的幾個(gè)特性!
觸摸屏的第一個(gè)特性:透明,它直接影響到觸摸屏的視覺(jué)效果。透明有透明的程度問(wèn)題,紅外線(xiàn)技術(shù)觸摸屏和表面聲波觸摸屏只隔了一層純玻璃,透明可算佼佼者,其它觸摸屏這點(diǎn)就要好好推敲一番,“透明”,在觸摸屏

-
29
2022-11英菲智科技容性液體檢測(cè)芯片深受客戶(hù)好評(píng)
英菲智科技的容性液體檢測(cè)芯片再經(jīng)過(guò)時(shí)間的考驗(yàn)后,獲得很多用戶(hù)的好評(píng)
-
11
2022-04英菲智科技推出DFN8封裝三通道觸摸芯片!
隨著部分電子產(chǎn)品對(duì)產(chǎn)品體積以及功耗要求越來(lái)越高,通常產(chǎn)品需要更小的體積以及更低的功耗以滿(mǎn)足產(chǎn)品越來(lái)越小型化低功耗的要求。英菲智科技有限公司為了滿(mǎn)足客戶(hù)的需求,推出了體積更小的3路觸摸對(duì)
-
26
2021-09英菲智科技喜遷新址
深圳市英菲智科技有限公司喜遷新址。英菲智科技根據(jù)業(yè)務(wù)發(fā)展需要,搬遷到新的辦公地址,歡迎新老客戶(hù)前來(lái)洽談!
-
04
2020-08英菲智科技推出單觸摸芯片144路觸摸應(yīng)用
深圳市英菲智科技有限公司一直以來(lái)致力于觸摸解決方案!
-
25
2019-11英菲智科技推出超強(qiáng)防水觸摸芯片
IFA1W01為強(qiáng)力防水性觸摸感應(yīng)芯片,外圍電路簡(jiǎn)單,應(yīng)用方便,可以應(yīng)該用在有防水需求的產(chǎn)品上。低功耗設(shè)計(jì)使得芯片可以應(yīng)用在電池供電的產(chǎn)品上。
-
10
2019-05深圳市英菲智科技隆重推出24通道觸摸芯片
深圳市英菲智科技近日推出集成24觸摸通道的觸摸MCU,LQFP64封裝, 芯片內(nèi)置2K字節(jié)的RAM, 提供8K,16K和32K 字節(jié)的FLASH的程序存儲(chǔ)空間供用戶(hù)選擇。芯片內(nèi)部集成了LED驅(qū)動(dòng)電路,最多可以驅(qū)動(dòng)8*32(CO